The Science Behind Our Recommendation Engine
Our Engagement Suite is designed to keep your visitors on your site longer and to keep them coming back. We can't make your content better -- that part is up to you. But when you have great content, we want to make sure that your visitors can discover it easily.
One of the most important features in Slickstream is what we call our "recommendation engine". This lives "in the cloud". We maintain a complete model of all of the content on your site. And we watch every pageview and every click by every visitor to your site. We use all of that data to make predictions about what content to bring to the attention of your visitors. That's what the recommendation engine does.
Slickstream widgets are the means by which we use that data to present content discovery opportunities to your visitors. Our primary widgets supporting recommendations are our "filmstrips". These are widgets that we inject into your pages that make it easy for visitors to see other things on your site that may interest them.
Let's start at the "top" and work our way down.
Here's a look at a filmstrip when we inject it inline near the top of a page. The filmstrip can go anywhere (and will adapt to the space it is given). But we find that it works best near (usually below) your banner and above the content. This is where people naturally go when they are thinking about navigation. Also, before they get into the details of the content, it reminds them that there are other opportunities waiting for them when they finish here.
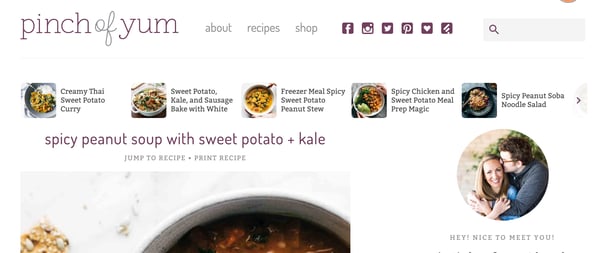
The filmstrip is highly configurable. Depending on the site and images, we may use a variety of different presentations. For example, here's another example of a filmstrip on a site with images that contain their own text:
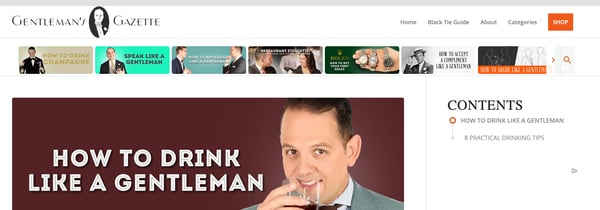
This first filmstrip appears inline in your content. As the viewer scrolls down the page, it scrolls off along with your content.
We realized that when visitors are starting to finish with your content, they usually start scrolling back to the top of the page. This is a great opportunity to grab them before they leave your page. So we invented the "filmstrip toolbar" widget. This is a "sister" to the filmstrip widget. It looks similar (although not identical because of where it appears) but is attached to the top of the screen (although can be linked to an existing sticky banner if you have one). You can decide whether you'd like it stuck to the top of the page all the time -- although we usually have it appear only when the user is scrolling backward toward the top of the page. Here's what it looks like:

Notice that these filmstrips are scrollable. Using a gesture, visitors can find more and more content available there. We call it "infinite scrolling" because the more they scroll, the more content they'll find until they've reached almost everything on your site!
In this example, you also see a search button on the right edge of the filmstrip. That is an option for visitors who might start asking if something specific is available here on the site. This opens our search panel where you can quickly find something specific you are looking for.
Okay. That's what filmstrips look like. But what content is it recommending? Is it always the same? Does it change over time? Let's talk about the recommendation engine.
The recommendation engine lives in the Slickstream cloud. It is like a living breathing entity. It is constantly taking in new information and it is learning about which recommendations work better and worse in different situations. If you keep refreshing the same page, you will probably see the recommendations changing. And certainly as you visit different pages, you'll see different recommendations on each one. How is it deciding?
We start with a map of your site's content. Think about each post like a city on the map. We arrange the map with connections (like "roads") connecting the cities. We are trying to design the map so that cities with things in common tend to be near to each other. We talk about the "affinity" between two posts as a measure of how related they are. After studying a lot of data, we've learned that the most important determinant of a good recommendation is this similarity. If you're looking at a recipe for a gorgeous chocolate cake, the world's most popular recipe for smoked salmon may not be the best recommendation. It's much more likely that a visitor on that page will choose another enticing chocolate dessert.
Having said that, we've also learned that visitors' behavior can tell us a lot. Our filmstrips present many possibilities to choose from. As visitors make choices, we are paying attention. If we see lots and lots of people in Boston choosing to visit Minneapolis next, we know we should be adjusting our map accordingly -- moving Boston closer to Minneapolis. We do this carefully, but over time, the map adjusts slowly in response to this data.
So you can expect that clickthrough rates with our filmstrips are going to slowly get better and better over weeks or months depending on how much traffic your site gets. That's our goal: to make small steady progress forward on viewer satisfaction.
What happens when you post some new content? Does that mean that we start all over again from scratch? Of course not. We find out immediately when that new content goes live. Our first move is to decide where to place it on our map. We use information about the content (like its title, description, categories, tags, etc.) to decide where to place it on our map. We choose the closest "cities" as its initial set of recommendations. And then we let the map continue to evolve from there. This means that this new content immediately has some good recommendations that will start getting better. And some of the old content that is nearby now has another candidate to include within its own set of recommendations.
Compare this to other "related post" plugins that you might have used. We think you'll find that better recommendations make a big difference in terms of usage.
Think of our filmstrips as the "face" and the recommendation engine as the "brains". It's a great combination.